The Intelligent Maintenance Conference is an international event on new diagnostic and intelligent monitoring methods for industrial systems! Our objective is to be a place to share fruitful discussions on predictive maintenance between experts from the industry and the academic world. Renowned speakers from varied backgrounds, including railway, healthcare, energy, and chemical industries, academics, and others, will be present.
We are excited to announce that, for the first time, the 8th Intelligent Maintenance Conference will include a dedicated 1-day workshop on the latest advancements and practical applications of artificial intelligence in maintenance technology.
Confirmed talks
Biologically-inspired Design and Control of Robots for Agile Flight and Locomotion
Prof. Dario Floreano
Laboratory of Intelligent Systems, EPFL
Animals display compliance, agility, and adaptability to a range of environmental situations that are still challenging for today's robots. In this talk I will describe the translation of biological principles of collision resilience, variable stiffness, morphological adaptation and multi-agent coordination into robots that could be deployed for inspection missions in challenging situations.
Responsible AI Development and Legal Compliance: Navigating the New Landscape of AI Regulation
Dr. Rialda Spahic
Responsible AI, Equinor
Responsible AI algorithms are crucial for organizations to ensure AI adoption is aligned with ethical standards and societal values, while enhancing trust and emphasizing safety in AI systems. With the upcoming EU AI Act, organizations are facing new legal obligations centered around risk management, data governance, bias mitigation, and accountability, with an emphasis on high-risk use cases. Therefore, it is crucial to examine the best practices and guidelines on designing and adopting responsible AI algorithms through ethical considerations, technical best practices, and regulatory compliance.
Leveraging AI and Non-Intrusive Monitoring as Intelligent Maintenance Enablers for Electromechanical Systems
Dr. Javier Diaz-Rozo
Aingura IIoT
This presentation outlines the integration of novel non-intrusive monitoring techniques with cutting-edge probabilistic machine learning advancements, establishing a robust and scalable intelligent maintenance approach for electromechanical systems. Through the analysis of two real-world case studies involving high-capacity water pumps and airport baggage inspection systems, the efficacy of this approach is demonstrated in not only detecting and predicting failures but also providing comprehensive diagnostic capabilities through explainability methodologies. Thus, a holistic methodology is showcased, from data analysis to actionable insight generation.
Potential Impact of Causal Inference on Railway Predictive Maintenance
Alexandre Trilla
Alstom
The technical field of Predictive Maintenance leverages data science to maximize the availability of industrial assets through their degradation models. In this sense, Artificial Intelligence and Machine Learning have proven to be effective techniques for extracting latent patterns from the available data. However, the observed data may sometimes be incomplete and misleading because real-world condition monitoring records rarely exhibit all of the operating regimes and wear rates of the machines. Therefore, to increase the reliability of the Predictive Maintenance solutions in a scenario where the behavior patterns change, Causal Inference emerges as an avenue of improvement by elegantly blending data-driven and expert-driven criteria to ultimately help maintainers make better informed decisions to manage their assets. In this talk, we review the potential of Causal Inference for Predictive Maintenance through two specific case-studies.
Predictive maintenance for heavy duty vehicles
Prof. Sepideh Pashami
Halmstad University
Predictive maintenance for heavy duty vehicles, like any other equipment, aims at predicting failures by analyzing sensor data. Yet the unique nature of heavy-duty vehicles operating in uncontrolled environment poses novel challenges in effectively detecting symptoms of impending issues. AI has been shown to provide accurate detection and identification of deviations from typical behavior. The question now is that how to proceed with flexible, robust, understandable and scalable solutions. This talk describes the application area and presents implementation of domain adaptation and explainability methods to address some of the existing challenges.
Accelerating Production Ramp-ups with a Single Pane of Glass for Production Data
Mathias Pawlowsky
Planted Foods
Plant-based meat is a rapidly growing industry, driven by the increasing demand from consumers seeking to reduce their animal meat consumption without sacrifice. In this highly competitive market, the speed of product innovation and subsequent production ramp-up is crucial. This talk showcases how Planted combines disparate production data sources into a single pane of glass to enable better and faster decisions during ramp-ups. You will hear how the production team monitors machines and runs root cause analysis on the process data, and how insights from the process data translate to improved control of the machines.
eXplainable Artificial Intelligence (XAI) in Industrial Diagnostic: Interpretable Unsupervised Anomaly Detection
Prof. Gian Antonio Susto
University of Padova
Unsupervised learning approaches have strong applicability in many scenarios, given the unavailability of labeled data in many settings. In particular, anomaly detection approaches have become paramount for ensuring the monitoring of critical systems. This talk delves into eXplainable Artificial Intelligence (XAI) in anomaly detection, with reference to an innovative approach called ‘Interpretable Anomaly Detection with DIFFI: Depth-based Feature Importance of Isolation Forest’. Moreover, in the talk, some applications to industrial use cases will be discussed, and other directions for research in anomaly detection and XAI will be outlined.
Crafting the Unseen: Generating Anomalies Solely from Descriptions
Han Sun
IMOS, EPFL
Fault detection is a crucial task across different application fields and involves different data types. If not just the detection of a fault is required but also the ability to distinguish between different fault types, unsupervised methods are not sufficient and supervised methods not only suffer from imbalance but also specialize on faults that have been already observed before. Given the rarity of faults, it is unrealistic to assume that all fault types have been observed within a short period of time during training dataset observation period. However, we typically can assume that domain have knowledge on the expected fault types (e.g. through a failure mode and effect analysis). Recently, the introduction of Large Language Models (LLMs) has opened new possibilities across various domains, including industrial operation and maintenance. This progress enables integration of semantic domain expert knowledge to anticipate and describe possible anomalies and faults also for new fault types that have not been observed before. Leveraging the expertise knowledge, our recent work proposes a novel approach to transform expert descriptions of fault types into guidance for realistic anomaly generation. By combining LLMs and diffusion models, we are able to generate realistic, diverse and semantically meaningful anomaly samples. By doing so, we not only enhance the interpretability of generated anomalies but also improve the performance of downstream anomaly detection tasks. This talk will give insights in our proposed framework, showcasing its advantages over traditional unsupervised anomaly detection and generation techniques. Furthermore, it will discuss the potential of LLMs for enhancing industrial fault detection, highlighting their promising performance in generic anomaly generation and understanding.
A Novel Physics-informed Neural Networks Approach for Efficient Multimodal Mapping and Inversion of Vibrations
Dr. Saeid Hedayatrasa
Flanders Make-MotionS
The vibrational characteristics of structural components contain valuable insights into their inherent mechanical properties and overall health status. Consequently, there's a pressing demand for efficient physics-based inversion algorithms. These algorithms must effectively reconstruct responses at unmeasured locations and/or identify unknown mechanical properties using a sparse set of noisy sensory data. Physics-informed neural networks (PINNs) offer promising solutions by seamlessly integrating the governing physics equations into their framework. Nonetheless, challenges arise due to the complex, multimodal, and multiscale nature of vibrational responses, along with the spectral bias of PINNs. In this talk we present a novel PINNs approach for efficient multimodal mapping of vibrations in a composite laminate and identification of its orthotropic elastic properties, given limited number of noisy simulation data points.
Modeling Multi-Body Dynamical System: Graph Neural Networks with Physics-Informed Inductive Biases
Vinay Sharma
IMOS, EPFL
Multi-body dynamical systems like bearings, gearboxes, and suspension systems are crucial across industries. Developing digital twins for these enables early fault detection and efficient maintenance, requiring real-time modeling of system dynamics for varied conditions and inputs. This talk presents an innovative Graph Neural Network (GNN) approach, integrating physics-informed inductive biases, for efficient real-time modeling. Our modular GNN framework treats system components as nodes and interactions as edges, facilitating direct learning of dynamics from trajectory data. This method not only improves modeling accuracy but also aids in parameter inference, enhancing fault analysis and system understanding. We highlight the model’s accurate dynamic predictions, particularly for bearings.
Industrializing the Automated Condition Monitoring and Predictive Maintenance of Pantograph Collector Strips
Urs Gehrig
SBB
Predictive maintenance driven by automated condition monitoring offers substantial potential for optimizing maintenance processes and increasing efficiency. At the Swiss Federal Railways (SBB), we have industrialized an innovative solution called the "Panto-Scanner" that utilizes YOLO and DeepLab algorithms to automatically extract pantograph collector strips from images. It then measures the strip thickness, which follows a typical wear and tear deterioration pattern, enabling accurate prediction of remaining useful life. This talk will present the successful implementation journey of the Panto-Scanner from proof-of-concept to a fully integrated predictive maintenance application within SBB's processes. Insights will be shared on leveraging automated condition data, data processing, and prognostics to improve maintenance planning, resource allocation, and decision-making. Real-world benefits achieved, including enhanced efficiency, reduced downtime, and improved safety, will be discussed. Additionally, perspectives on the interdisciplinary collaboration between railway operators, infrastructure providers, suppliers, and academics will be provided.
Intelligence In Maintenance—For Whom, Doing What?
Henrik Göthberg1, Mikael Klingvall2
1CEO DAIRDUX, 2 DAIRDUX
It's not about data or AI. It's about the productivity of data workers and the productivity of those that consume data work. The current Data & AI narrative risks being an anti-pattern, creating a blind spot that inhibits productivity gains. We want to flip the script on how and why we do data work and employ AI in data-intensive operations. We propose a fundamentally agent-based perspective where actual data workers, AI or human, transform data into something intelligent—useful and adoptable—for people solving problems. We are on the verge of deploying autonomous AI agents—a new era for organising and operating our enterprises. This keynote offers new semantics that address the sociotechnical complexity of AI and data work and the cross-functional teams that make products for data workers or maintenance operations.
Strategic Growth and Value Creation: Excellence through benchmarking and exchange beyond company’s boarders in MedTech
Ralph Fasler
Vice President Manufacturing, Ypsomed AG
This talk will provide insights into how the value creation processes at Ypsomed can keep pace with the strong growth of the MedTech company. A focus will be placed on the regular direct benchmarking and exchange with other hidden champions from various industries with similar requirements and characteristics. This makes it possible to compare and continuously improve outside of competitive situations.
Predictive analysis of railway asset regeneration
Dr. Amira Youssef
SNCF
The decision-making tool currently under optimisation aims to anticipate the regeneration needs of railway assets. These forecasts are based on multi-criteria optimization algorithms, considering various constraints, particularly budgetary and ressources availabities ones. Optimization criteria are based on statistical studies such as survival analysis, maintenance costs versus regeneration costs, and impacts on regularity in case of incident. This tool offers asset managers a well-informed perspective for planning interventions, optimizing the efficiency of installations by replacing them at the most opportune moment, and improving safety by reducing the risks associated with unexpected breakdowns.
Principles of Predictive Maintenance on aircraft
Vincent Cheriere
Airbus
The predictive maintenance on aircraft has become routine work in the organisation of pioneering innovative airlines today. This session will present the principles of predictive maintenance and the successive decisions that Airline Engineers take to predict failures and order predictive equipment removals on the right time. The speaker will address the multiple parameters taken into account in decision-making regarding predictive removal, the supply of spare parts, verification of performance and confirmation of the correct decision a posteriori.
Asset Management in the Digital Age
Prof. Ajith Parlikad
University of Cambridge
The practice of maintenance and asset management has the potential to be one of the biggest beneficiaries of technologies such as Industrial Cyber Physical Systems and Digital Twins. Supported by new data analytics and innovative machine learning techniques, the link between the physical assets and their virtual counterpart can allow optimised maintenance and operation of industrial systems. Although this presents major opportunities for companies in the manufacturing and infrastructure sectors to improve their offerings, there are some key challenges to be addressed. This talk – structured around our current research – will explore the opportunities and challenges presented by the emerging digital technologies and techniques on the management of complex assets and systems.
Topic will be available soon
Jose Celaya
SLB
The full program will be available soon.
If you would like to become a sponsor please contact us using the form below or by .
IMC Hands-on Workshop
Join us at the 8th Intelligent Maintenance Conference for the interactive IMC Hands-on Workshop (IMC HOW?). We are excited to announce that, for the first time, the 8th Intelligent Maintenance Conference will include a dedicated 1-day workshop on the latest advancements and practical applications of artificial intelligence in maintenance technology. The workshop will cover the following topics:
The Power of Graph Neural Networks: A Comprehensive Introduction and Industrial Applications
Given by Mengjie Zhao and Raffael Theiler
IMOS, EPFL
Graph Neural Networks (GNNs) offer a powerful way to analyze complex assets and systems. They have been applied to a wide range of industrial applications, from the analysis of individual components such as bearings or turbofan engines to condition monitoring of large-scale interconnected systems such as power plants, distributed heating networks or energy distribution networks. In this workshop, we start by exploring the theoretical basics of how Graph Neural Networks (GNNs) facilitate learning in data that is structured as entities and their relationships. Then we'll apply GNNs to industrial applications that benefit from this relational interpretation of the data and learn how GNNs enable model generalization to unseen environments. The workshop includes a comprehensive introduction to GNNs followed by a hands-on tutorial using an industrial dataset, giving you direct guidance in applying these techniques to solve practical problems.
Mastering Domain Adaptation in Deep Learning: A Practical Workshop
Given by Han Sun
IMOS, EPFL
In the dynamic field of Deep Learning (DL), the ability to adapt models to new, unseen domains is crucial for achieving robust and generalizable performance across a wide range of applications. Designed for practitioners and researchers with a basic understanding of deep learning, this hands-on workshop is designed to introduce participants to the concept and techniques of domain adaptation in DL, providing them with the practical skills and knowledge needed to navigate and implement these strategies effectively.
Understanding your Deep Learning Models with Explainable AI
Given by Dr. Florent Forest
IMOS, EPFL
This workshop offers a practical introduction to various eXplainable artificial intelligence (XAI) tools that can be leveraged, on the one hand, to explain the decisions of already trained deep neural networks, or on the other hand, to make their architecture inherently more interpretable, with the ultimate goal of increasing trust and acceptance of AI-based systems. During this workshop, you will have the occasion to open the black-box of several models in industry-relevant applications.
Prerequisites:
- Beginner to intermediate experience in Python.
- A basic understanding of deep learning, basic knowledge of data science, and time series analysis will be helpful for understanding the theoretical background.
- A laptop with a stable internet connection and a Gmail account to be able to follow along during the hands-on sessions in Google Colab.
Registration
The early bird registration is open till June
30, 2024.
It is possible to book tickets for a single conference day.
*Students will be asked to show their student card at the conference and the workshop check-in. Workshop will take place only with enough attendees and is limited to maximum 30 attendees. You will be informed beginning of August if the event will take place. In case of cancellation, you will get reimbursed. Participants are asked to bring their own laptop to the workshop. Participants will receive an IMOS certificate of attendance on successful completion.
VENUE
Le Polydôme
EPFL, CH-1015 Lausanne
Our team
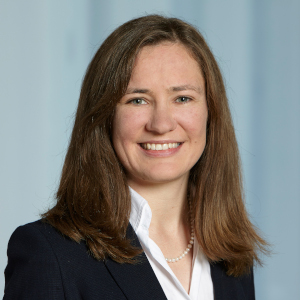
Prof. Olga Fink
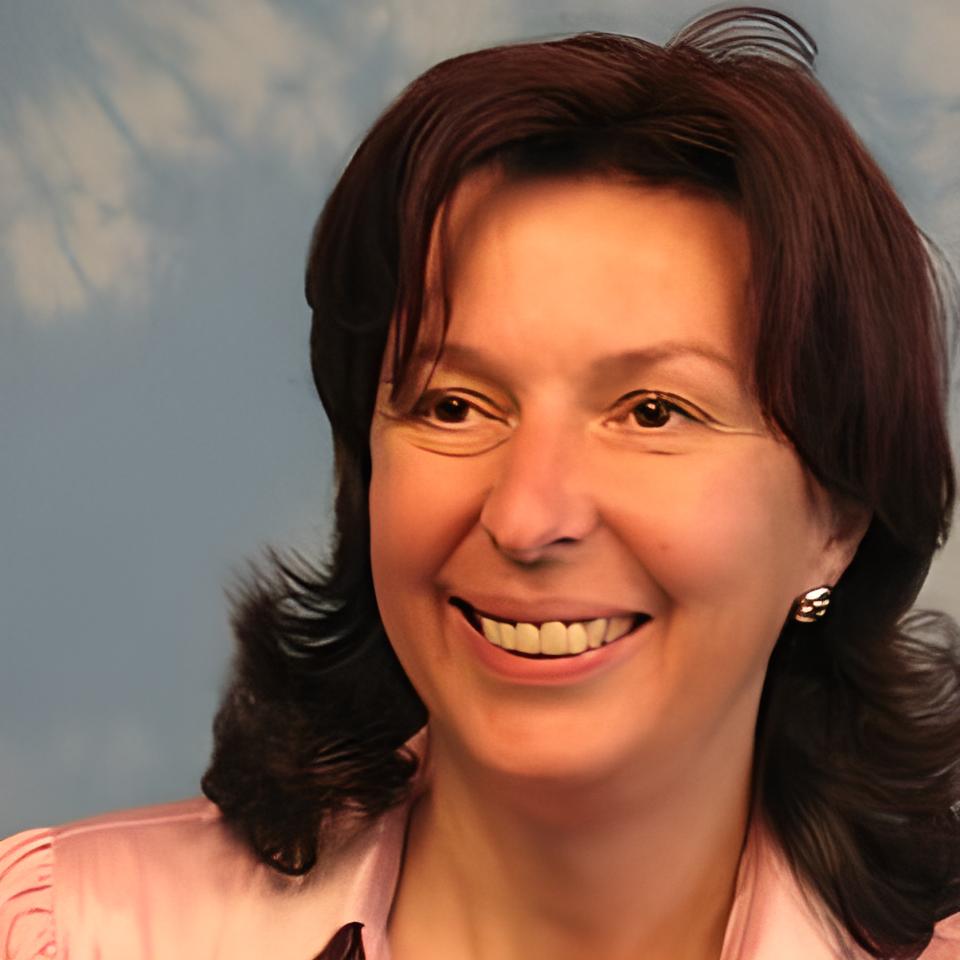
Christine Gabriel
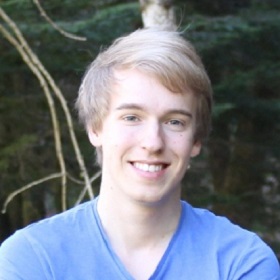
Dr. Florent Forest
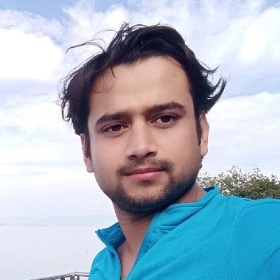
Vinay Sharma
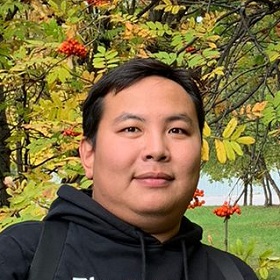
Sergei Garmaev
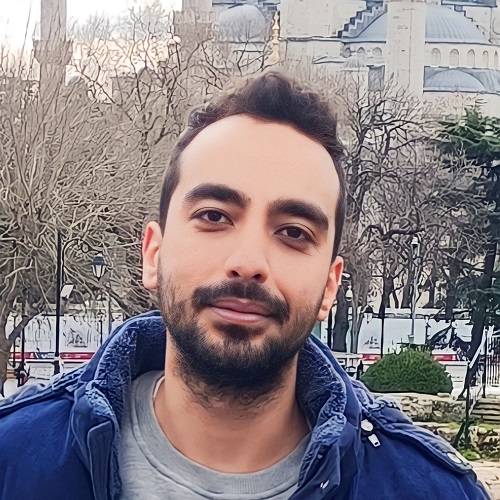
Keivan Faghih Niresi
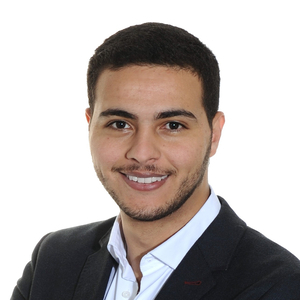
Ismail Nejjar
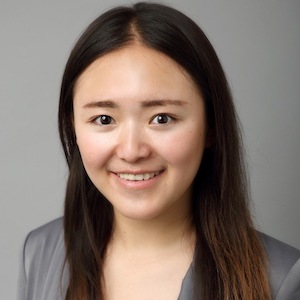
Mengjie Zhao
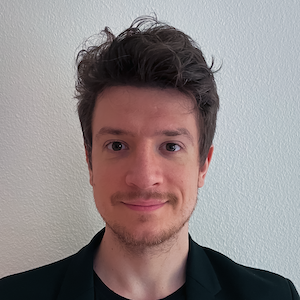
Raffael Theiler
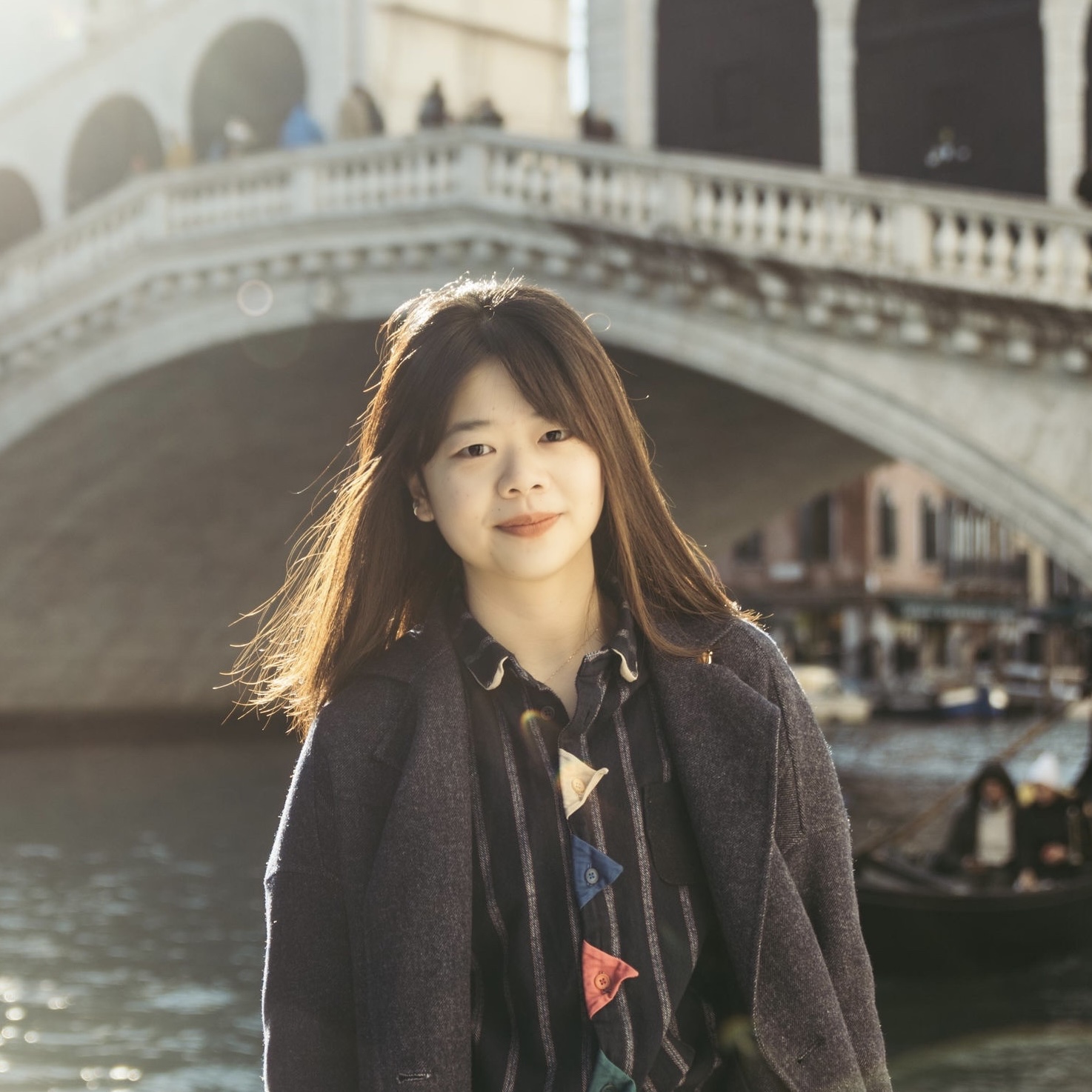
Han Sun
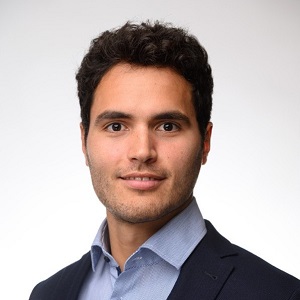
Leandro Von Krannichfeldt